🔍 Cos’è il Catastrophic Forgetting?
Il catastrophic forgetting (in italiano: oblio catastrofico o interferenza catastrofica) è un fenomeno che si verifica nei modelli di apprendimento automatico, in particolare nelle reti neurali artificiali, quando vengono addestrati sequenzialmente su più compiti. In pratica, il modello “dimentica” drasticamente le informazioni apprese in passato non appena impara qualcosa di nuovo (Wikipedia).
Ad esempio, se una rete neurale apprende a riconoscere gatti e successivamente viene addestrata a riconoscere cani, potrebbe perdere la capacità di riconoscere i gatti.
❝L’oblio catastrofico è una delle principali sfide nell’ambito del continual learning, ovvero l’apprendimento continuo❞ (Humai).
🧠 Perché succede?
Le reti neurali aggiornano i propri pesi sinaptici durante l’addestramento, e questi pesi sono condivisi tra tutti i compiti. Quando si addestra un nuovo task, l’ottimizzazione tramite backpropagation può sovrascrivere pesi cruciali per i compiti precedenti.
Questo comportamento è molto diverso dal cervello umano, che riesce a integrare nuova conoscenza senza compromettere quella esistente.
🔄 Strategie per Mitigare il Catastrophic Forgetting
Numerosi approcci sono stati sviluppati per affrontare il problema. Ecco i principali:
1. Replay e Rehearsal
- Utilizzano dati reali o sintetici dei compiti precedenti per rinfrescare la memoria del modello.
- Tecniche come il generative replay simulano dati vecchi tramite modelli generativi.
- Pro: Efficaci nel mantenere la performance.
- Contro: Aumentano l’uso di memoria e violano la privacy in certi contesti (Nightfall AI).
2. Regularizzazione
- Metodi come Elastic Weight Consolidation (EWC) penalizzano i cambiamenti drastici nei pesi importanti.
- Altri esempi includono Synaptic Intelligence (SI) e Memory Aware Synapses (MAS).
- Questi metodi introducono un termine di regolarizzazione nella funzione di perdita che conserva le conoscenze chiave (Medium).
3. Modifiche Architetturali
- Progressive Neural Networks: nuove colonne di rete per ogni compito, con connessioni laterali ai moduli precedenti.
- PackNet e PathNet bloccano e congelano parti della rete già apprese.
- Pro: Separazione netta tra i compiti.
- Contro: Scalabilità limitata (Jelvix).
4. Meta-Learning
- Tecniche come Model-Agnostic Meta-Learning (MAML) allenano il modello a imparare rapidamente nuovi task con minimo impatto sulle conoscenze precedenti.
- Spesso usate in scenari di few-shot learning o continual learning avanzato (LinkedIn).
📊 Settori colpiti
Il catastrophic forgetting ha implicazioni critiche in:
- Robotica: robot che devono adattarsi continuamente a nuovi ambienti.
- Medicina: modelli clinici aggiornati con nuove malattie.
- Sicurezza: sistemi di videosorveglianza che imparano nuovi pattern senza dimenticare quelli vecchi (Intelex Vision).
📌 Tabella di riepilogo delle soluzioni
Tecnica | Descrizione | Vantaggi | Svantaggi |
---|---|---|---|
Replay | Riutilizza dati passati | Facile, efficace | Uso memoria, problemi privacy |
Regularizzazione | Blocca cambi pesi importanti | Poco costosa | Rischia di limitare l’apprendimento |
Architettura dinamica | Divide la rete tra i compiti | Alta performance | Poco scalabile |
Meta-learning | Apprende ad apprendere | Adattivo, flessibile | Complesso, computazionalmente costoso |
🔮 Conclusione
Il catastrophic forgetting è una barriera significativa per lo sviluppo di sistemi di IA più intelligenti, continui e adattivi, simili al cervello umano. La ricerca in questo campo è in rapido sviluppo e combinare più approcci potrebbe essere la strada verso il lifelong learning.
✅ Le sfide sono grandi, ma anche le opportunità: costruire AI che ricorda, impara e si adatta nel tempo sarà cruciale per il futuro dell’innovazione.
🔗 Fonti
- Humai.it – Catastrophic Forgetting
- Wikipedia – Interferenza Catastrofica
- Nightfall AI – Replay Techniques
- Medium – Strategie di Mitigazione
- Jelvix – Why It Matters
- Intelex Vision – Effetti pratici
- LinkedIn – Concetto di Catastrophic Forgetting
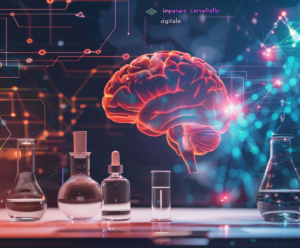
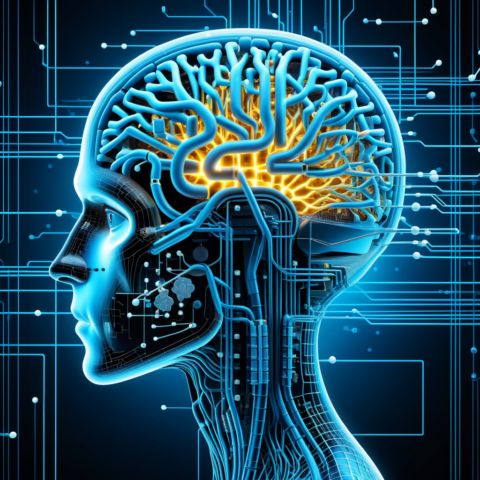
Affrontare il Catastrophic Forgetting: Strategie per l’Apprendimento delle AI
In questo episodio, esploriamo il fenomeno del Catastrophic Forgetting nelle reti neurali artificiali, analizzando le sue cause e le sfide che pone allo sviluppo di sistemi di intelligenza artificiale. Discutiamo le strategie innovative per mitigare questo problema, come il replay dei dati, la regularizzazione, le modifiche architetturali e il meta-learning, evidenziando l’importanza di costruire AI capaci di ricordare e adattarsi nel tempo per il futuro dell’innovazione.
Ascolta sAffrontare il Catastrophic Forgetting: Strategie per l’Apprendimento delle AI
In questo episodio, esploriamo il fenomeno del Catastrophic Forgetting nelle reti neurali artificiali, analizzando le sue cause e le sfide che pone allo sviluppo di sistemi di intelligenza artificiale. Discutiamo le strategie innovative per mitigare questo problema, come il replay dei dati, la regularizzazione, le modifiche architetturali e il meta-learning, evidenziando l’importanza di costruire AI capaci di ricordare e adattarsi nel tempo per il futuro dell’innovazione.
Script del podcast
Giulia
Benvenuti a tutti! Oggi parleremo di un argomento davvero affascinante e, oserei dire, cruciale per il futuro della tecnologia: il Catastrophic Forgetting nell’intelligenza artificiale. Sì, avete sentito bene! Ma che cos’è esattamente? Immaginate di studiare per un esame e, mentre imparate nuove informazioni, vi dimenticate di quelle che avevate già appreso. Questo è il problema che affligge i modelli di apprendimento automatico, in particolare le reti neurali artificiali. Quando addestrati su nuovi compiti, questi modelli ‘dimenticano’ drasticamente le informazioni precedenti. È un po’ come se ogni volta che imparassimo a suonare un nuovo strumento, ci dimenticassimo delle melodie che abbiamo appena imparato con il primo, vero?
Luca
Umm, aspetta un attimo! Quindi, stai dicendo che le AI possono ‘dimenticare’ le informazioni come noi umani? Cioè, è un po’… confuso? Per esempio, se un robot ha appena imparato a camminare ma poi inizia a imparare a danzare, dimenticherà come camminare? [sigh]
Giulia
Esattamente! È proprio così. E questa ‘dimenticanza’ avviene perché le reti neurali aggiornano i loro pesi sinaptici in modo tale che quando apprendono un nuovo compito, le informazioni precedenti possono essere sovrascritte. Pensala come se stessimo ristrutturando una casa: se mettiamo giù una nuova parete in una stanza, potremmo dover demolire parte della parete che esisteva precedentemente per fare spazio.
Luca
Oh, wow! Quindi le AI hanno come una sorta di ‘casa’ mentale che stanno sempre ristrutturando? Ma… ci sono dei modi per evitare che questo accada? Cioè, ci sono strategie, giusto? [laughs]
Giulia
Sì, ci sono diverse strategie! Ad esempio, il replay e il rehearsal, che coinvolgono la riutilizzazione di dati da compiti precedenti per rinfrescare la memoria del modello. Un po’ come quando studiamo rivedendo i nostri appunti per non dimenticare le cose!
Luca
Hmm, interessante! Ma, aspetta un momento. Ma se qualcuno studia usando solo i suoi appunti e non fa pratica, potrebbe dimenticare tutto lo stesso, giusto? È complicato, non credi? [sigh]
Giulia
Esattamente, è proprio così! Ecco perché è importante combinare vari approcci per affrontare il Catastrophic Forgetting. Altre strategie includono la regularizzazione, che penalizza i cambiamenti drastici nei pesi importanti, e le modifiche architetturali, che aggiungono nuove colonne di rete per ogni compito. È come avere diverse stanze nella casa per vari scopi!
Luca
Uuuuh! Come un albergo! Ogni stanza per un diverso tipo di ospite, giusto? Cioè, quindi le AI devono avere questo spazio per evitare confusione! Ma in quali settori vedremo concretamente effetti del Catastrophic Forgetting? [laughs]
Giulia
Ottima domanda! Settori come robotica, medicina e sicurezza sono molto colpiti da questo fenomeno. Immagina un robot chirurgo che deve imparare diverse tecniche operatorie. Se inizia a dimenticare procedure precedenti, potrebbe costare la vita a qualcuno—è una questione seria!
Luca
Oh mio Dio, questo è davvero inquietante! Quindi, ci sono ricerche attive per risolvere questo problema? Cioè, siamo a buon punto per creare AI che possa ‘ricordare’ come noi? [sigh]
Giulia
Sì, ci sono molti progressi in questo campo! La combinazione di vari approcci sta aprendo porte a sistemi di IA più intelligenti e adattivi. Il Catastrophic Forgetting è una barriera significativa, ma la ricerca è in rapida evoluzione. Immagina un AI che non solo ricorda, ma impara e si adatta nel tempo! Questo sarà cruciale per l’innovazione futura.
Luca
Uhm, mi sento quasi ispirato! È come se stessimo parlando dei superpotere delle macchine! Pensa se potessero ricordare come i nostri nonni! Che cosa incredibile! [laughs]
Giulia
Esattamente! Ecco perché il nostro lavoro è così importante. Costruire AI che non solo impara ma si evolve con noi, è il futuro verso cui stiamo andando. Quindi, rimanete sintonizzati! Le opportunità sono enormi e affascinanti!
Luca
Wow! Quindi fondamentalmente, stiamo progettando delle intelligenze artificiali che potrebbero diventare più sagge con il tempo? Che strano pensiero! [sigh]
Giulia
Sì, ed è proprio questo che rende tutto così entusiasmante! Stiamo davvero entrando in un’era in cui la tecnologia può non solo assistere ma anche imparare e crescere come noi!
Luca
Che affascinante! Immagina se un giorno l’IA potesse anche raccontare barzellette o fare arte! Oh, wow! [laughs]